Introduction
The liver is a critical organ for the body and it is known as the largest vital organ of the body [
1]. Liver diseases are very common in today’s society and require clinical care by an experienced doctor or nurse in all cases [
2]. Advanced chronic liver diseases, such as chronic failure, metabolic liver diseases, primary biliary cirrhosis, sclerosing cholangitis, biliary atresia, liver disease caused by alcohol and drugs and liver malignancies cause irreversible liver dysfunction [
3].
The last method of treating liver patients is liver transplantation, which has a high-risk percentage [
4, 5]. Liver transplantation is performed in many countries, the United States being the first [
6]. Namazi Hospital in Shiraz City is the leader in liver transplantation in Iran [
7]. The occurrence of complications after liver transplantation increases the hospitalization time of patients increases the difficulty of nursing and hospitalization costs [
8, 9]. Cognitive impairment, post-liver transplant encephalopathy, is a common complication after liver transplantation [
10]. In hepatic encephalopathy, due to the inability of the liver to detoxify, the concentration of toxic substances, including ammonia, increases in the blood and brain [
11, 12, 13]. On the other hand, an increase in ammonia also stimulates immune cells in the brain and causes neuroinflammation [
14]. High levels of ammonia and neuro-inflammation caused by it cause changes in blood transfusion levels, which in turn causes cognitive disorders, such as learning and memory deficits, as well as disturbances in movements and motor coordination [
15]. After transplantation, hepatic encephalopathy is largely responsible for avoidable medical complications and deaths globally [
16]. We can take proactive steps to lower the occurrence of encephalopathy if we can accurately forecast the danger of the condition. By lowering the likelihood of complications and indirectly lowering medical costs, post-surgical complications analysis can provide doctors with preoperative intervention indicators. To provide patients with an accurate prognosis and make plans for any encephalopathy that may occur [
17].
It is critical to stress that exercise-related knowledge in hepatic encephalopathy is currently restricted and in its early stages. In recent years, society has shown an increasing interest in health through physical activity and sports. Promoting physical exercise is essential in the prevention and promotion of well-being among the healthy population and in groups or patients with some kind of special needs (such as liver transplant recipients) [
18]. For individuals undergoing transplants, weight gain and elevated cholesterol are two prevalent chronic issues. The immunosuppressive medication and increased hunger are to blame for this. Numerous medical disorders, including metabolic syndrome (which is closely linked to increasing body fat around the waist and high blood levels of triglycerides and cholesterol), can be brought on by eating too much and exercising too little [
19].
Today, despite a large amount of medical data, diagnosing and predicting diseases is a difficult task, but with the introduction of computer science in the field of medicine, this problem has been solved to a large extent and researchers have been able to solve the challenges by introducing new methods. For solving the problem, one of these methods for predicting diseases is data mining and the use of machine learning methods [
20]. Machine learning methods have been used to predict complications after liver transplantation. Moghadam et al. (2020) using machine learning, showed a correlation between the occurrence of episodes of hypertension during or after surgery and several subsequent outcomes [
21]. Meanwhile, Pepin et al. (2020) created a model for predicting complications from laparoscopic hysterectomy surgery in recognizing benign conditions [
22]. Merath et al. (2020) developed a machine learning model and showed that these algorithms can be used for the electronic monitoring of patients with postoperative complications [
23]. Zeng et al. (2021) compared eight different machine learning methods and showed that machine learning-adaptive augmentation performed best in detecting surgical complications [
24]. Such studies have shown that machine learning can significantly predict the occurrence of postoperative disease. However, few studies have used machine learning to predict hepatic encephalopathy after liver transplantation. Accordingly, this study compares and predicts hepatic encephalopathy complications in liver transplant patients using a random forest algorithm in active and inactive men.
Materials and Methods
The statistical population of this study was 852 patients. Among these people, 350 active (162 healthy and 188 with encephalopathy symptoms) and 402 inactive men (210 healthy and 192 with encephalopathy symptoms) were selected as the subjects of this study. These subjects had undergone a liver transplant at Taleghani Hospital in Tehran City, Iran, during 2001-2022 and had laboratory records in the computer archive files of that center. The laboratory files of the patients contained laboratory information before and after transplantation, personal information, and lifestyle. By examining these files, we gathered data on 28 characteristics, such as age, sex, duration of stay following surgery, etc. The findings of scholarly publications, as well as the advice of two highly qualified medical professionals in the field of liver transplantation, we performed a secondary screening of the variables and finally, 14 features were selected as input to the algorithm. Male gender, age between 40 and 75 years, hospitalized clinical trials and medical records, and phone or internet accessibility were among the inclusion criteria. Meanwhile, having a chronic illness, taking hormonal medications in the past, and receiving treatment for osteoporosis were among the exclusion criteria.
The characteristics that were selected included age between 40 and 75 years, height, weight, body mass index, alkaline phosphatase, alanine aminotransferase, aspartate aminotransferase, cholesterol, triglycerides, blood sugar, family history, fatty liver, liver cancer, and diabetes. At this stage, MATLAB version 2021 was used to import and analyze the Excel data collection.
Random forest data mining algorithm
In liver transplant patients, postoperative hepatic encephalopathy was predicted using the random forest data mining technique. It is a simple machine-learning method that frequently yields excellent results even when its meta-parameters are left alone. Because of its ease of use and simplicity, this method is among the most often used for both regression and classification. It is regarded as the most used algorithm for machine learning [
25].
When compared to many other methods, a random forest’s training period is quick. They can be used to estimate missing data and are resistant to overfitting. Due to its versatility, the technique can be applied to jobs involving both regression and classification [
26]. The primary tenet of this approach is that the sum of learning models improves the model’s overall performance. To put it simply, a random forest creates many decision trees and combines them to generate forecasts that are more reliable and accurate [
25].
When it is impossible to divide two classes of the same data using straight lines, this technique can be quite helpful (
Figure 1).
The performance of the algorithms was assessed using accuracy and precision. By dividing the total number of forecasts by the number of right predictions, the algorithm’s prediction accuracy (
Equation 1) is demonstrated. The algorithm’s accuracy demonstrates its capacity to distinguish between those who are healthy and those who have signs of encephalopathy. By dividing the total number of forecasts by the total number of predictions in each row, precision can be calculated (
Equation 2) [
26].
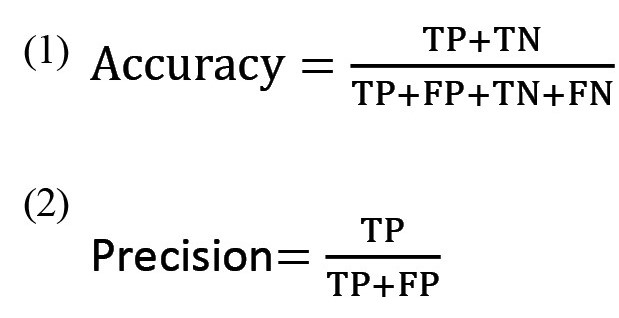
where, TP: True positive; TN: True negative; FP: False positive; FN: False negative.
The accuracy and precision standards according to the data evaluation methodology are displayed in
Table 1.
Results
Table 2 shows the anthropometric characteristics and
Table 3 shows the descriptive statistics related to the quantitative and qualitative variables of the subjects’ files.
Table 2 and
Table 3 present the factors that were determined using the data from scholarly journals and doctor questionnaires. Age, height, weight, body mass index, aspartate aminotransferase, alanine aminotransferase, alkaline phosphatase, triglyceride, cholesterol and blood sugar are quantitative and continuous variables, while family history, fatty liver, liver cancer, and diabetes are qualitative and discrete variables. Meanwhile, 70% of the data were used to train the algorithm, while 30% were used for testing. The random forest method was employed to forecast the consequences of encephalopathy.
Figure 2 displays the confusion matrix findings for this approach.
The results showed that the random forest algorithm can predict with 76.2% accuracy and 73.7% precision in active men with encephalopathy and without complications.
The results showed that the random forest algorithm can predict with 75.5% accuracy and 72.4% precision inactive men with encephalopathy and without complications.
Figure 3 shows the random forest algorithm's confusion matrix for inactive men.
Discussion
Liver transplantation represents the definitive and final treatment of liver disease in the final stage, and cognitive impairment follows repeated liver transplantation, which is called encephalopathy post-liver transplantation. In various studies under the title of medical data mining, several solutions have been presented, including the use of algorithms to discover the relationships between the factors of various diseases. Some data mining algorithms, such as machine learning methods, can predict different situations in the future by gradually learning these patterns and existing conditions, in addition to analyzing data and extracting hidden patterns in them [
27]. An issue that has not been addressed is the use of data mining in the prediction of diseases related to liver transplantation. This study compared and predicted hepatic encephalopathy complications in liver transplant patients using a random forest algorithm in active and inactive men. In this study, 30% of the data were considered for testing and 70% for training the algorithm. The results of this study revealed that the random forest algorithm using 14 recipient features has an accuracy of 76.2% in diagnosing hepatic encephalopathy after liver transplantation in active men and 75.5% in inactive men.
In line with the results of this study, Shahraki and Mesgar (2019) compared different data mining algorithms to predict liver diseases in their study. In their study, they used the most dangerous indicators of these diseases such as aspartate aminotransferase, gender, alkaline phosphatase, age, direct bilirubin, albumin, total bilirubin, the ratio of albumin to globulin and total protein for prediction and diagnosis and at the end of the algorithm They reported the enhanced decision tree with 94.04% accuracy as the best performance [
28]. Cruz-Ramírez et al. (2013) reviewed various machine-learning models for predicting patient survival after liver transplantation. In their study, they built models that predict whether a patient will receive an organ after liver transplantation in a certain time horizon [
29]. In this study, they used the observations of bilirubin and creatinine in the entire first year after transplantation to obtain predictors, and their static value and variability were obtained and examined. The models of these researchers have a high predictive power, which confirms the value of combining the diversity of biochemical measurements. They found that models with full complexity, such as random forests and gradient boosting, had the best predictive power. Fernández-Delgado et al. (2014) in a study titled, “Hepatic Encephalopathy After Liver Transplantation”, showed signs of altered consciousness in the presence of drowsiness, confusion, and tremors with an increase in serum ammonia (63 moll/L, normal value <50) due to obvious signs of acute heart failure and they reported a decrease in left ventricular ejection fraction to 20% in predicting hepatic encephalopathy [
30]. Random forest classifiers were determined to be the most accurate in a recent groundbreaking study that classified all 121 data sets, which represented the entirety of the University of California Irvine Machine Learning Repository, using the performance of 179 different machine-learning classifiers [
31].
In individuals who are in a stable clinical state, moderate physical activity could be recommended. In hepatic encephalopathy, an exercise regimen combined with the best possible diet may help to preserve or even grow muscle mass, preventing sarcopenia and frailty. Muscle mass could contribute to the development or aggravation of hepatic encephalopathy [
32].
However, the quality and quantity of muscle mass may determine how well it eliminates ammonia. Thus, increasing muscle mass and function could be a key tactic in reducing hepatic encephalopathy. Targeting the muscle with exercise could be an effective treatment method for lowering the risk of hepatic encephalopathy after liver transplantation. Exercise training is an intriguing, straightforward, and inexpensive approach that may alter muscle mass in hepatic encephalopathy. Exercise has been demonstrated to dramatically increase lean mass and strength in both people and healthy animal models by stimulating muscle satellite cell proliferation and activating protein synthesis [
33]. This implies that physical activity might be preventive against frailty, sarcopenia, and consequently hepatic encephalopathy, as well as a useful tool for managing these conditions. It has been demonstrated that in patients with hepatic encephalopathy, inactivity exacerbates muscular atrophy [
34].
Conclusion
This study demonstrates that a random forest method based on recipient factors may be used to predict hepatic encephalopathy problems. This technique might help transplant surgeons make better judgments about hepatic encephalopathy problems. The ability to quantify risk may allow for improved confidence and better outcomes after transplantation. Also, an activity may help prevent or attenuate complications of hepatic encephalopathy. It is suggested that this model be tested with more data sets and information on more healthy people.
Study limitations
One of the most important limitations of researchers in this field is the lack of sufficient data related to healthy people because, in most of the registration data, the number of samples of healthy people is much less than that of patients. The existence of this asymmetry can lead to the lack of complete learning of algorithms and reduce the accuracy of diagnosis. Another limitation of this algorithm is that although it has been trained to predict the post-transplant encephalopathy complication; however, other significant outcomes of liver transplantation, such as graft failure, dysfunction, acute or chronic rejection, infection, immunosuppression, or the absence of late biliary stricture, may have an impact on its predictive accuracy and may necessitate the use of a different training algorithm.
Considering the importance of liver diseases, it is necessary to identify a method to predict and diagnose these diseases in time. For further investigation in this field, data from other medical centers and other algorithms can be used in future studies and the results can be compared. It is also recommended to prepare the field of using these algorithms in the prediction of encephalopathy after liver transplantation in hospitals so that doctors and specialists can investigate and predict this liver complication using a suitable environment and thus compensate for the occurrence of injuries. It is not possible to prevent liver transplant patients. Finally, it is suggested to use other methods and algorithms to exploit human knowledge to create a prediction model.
Ethical Considerations
Compliance with ethical guidelines
The present study was conducted according to the Helsinki Ethics Statement, and also the Research Ethics Committee of Allameh Tabatabai University approved the study protocol (Code: IR.ATU.REC.1401.051). Participants entered the study after providing written informed permission and had the opportunity to withdraw at any time.
Funding
This research did not receive any grant from funding agencies in the public, commercial, or non-profit sectors.
Authors' contributions
All authors equally contributed to preparing this article.
Conflict of interest
The authors declared no conflict of interest.
Acknowledgments
The authors are grateful to all the participants in this study and all the people who accompanied us in conducting this research, as well as Taleghani Hospital.
References
- Wasityastuti W, Habib NA, Sari DCR, Arfian N. Effects of low and moderate treadmill exercise on liver of d-galactose-exposed aging rat model. Physiological Reports. 2019; 7(21):e14279. [DOI:10.14814/phy2.14279] [PMID] [PMCID]
- Winder GS, Fernandez AC, Klevering K, Mellinger JL. Confronting the crisis of comorbid alcohol use disorder and alcohol-related liver disease with a novel multidisciplinary clinic. Psychosomatics. 2020; 61(3):238-53. [DOI:10.1016/j.psym.2019.12.004] [PMID]
- Mantovani A, Scorletti E, Mosca A, Alisi A, Byrne CD, Targher G. Complications, morbidity and mortality of nonalcoholic fatty liver disease. Metabolism. 2020; 111S:154170. [DOI:10.1016/j.metabol.2020.154170] [PMID]
- Arab JP, Izzy M, Leggio L, Bataller R, Shah VH. Management of alcohol use disorder in patients with cirrhosis in the setting of liver transplantation. Nature Reviews. Gastroenterology & Hepatology. 2022; 19(1):45-59. [DOI:10.1038/s41575-021-00527-0] [PMID] [PMCID]
- Dolcet A, Heaton N. Paediatric Liver Transplantation. In: Guandalini S, Dhawan A, editors. Textbook of pediatric gastroenterology, hepatology and nutrition: A comprehensive guide to practice. London: Springer Nature; 2022. [DOI:10.1007/978-3-030-80068-0_76]
- Duchateau NM, Canovai E, Vianna RM, Vanuytsel T, Verslype C, Mazariegos GV, et al. Combined liver-intestinal and multivisceral transplantation for neuroendocrine tumors extending beyond the liver: A systematic literature review. Transplantation Reviews. 2022; 36(1):100678. [DOI:10.1016/j.trre.2022.100678] [PMID]
- Kentish-Barnes N, Cohen-Solal Z, Souppart V, Cheisson G, Joseph L, Martin-Lefèvre L, et al. Being convinced and taking responsibility: A qualitative study of family members' experience of organ donation decision and bereavement after brain death. Critical Care Medicine. 2019; 47(4):526-34. [DOI:10.1097/CCM.0000000000003616] [PMID]
- Tian M, Wang B, Xue Z, Dong D, Liu X, Wu R, et al. Telemedicine for follow-up management of patients after liver transplantation: Cohort study. JMIR Medical Informatics. 2021; 9(5):e27175. [DOI:10.2196/27175] [PMID] [PMCID]
- Chen C, Chen B, Yang J, Li X, Peng X, Feng Y, et al. Development and validation of a practical machine learning model to predict sepsis after liver transplantation. Annals of Medicine. 2023; 55(1):624-33. [DOI:10.1080/07853890.2023.2179104] [PMID] [PMCID]
- Khalid MB, Nagorna A, Rippel N, Ezaz G, Schiano TD, Crismale JF. Early neurologic complications after liver transplant are associated with reduced long-term survival and increased rates of rejection. Liver Transplantation. 2023; 29(10):1079-88. [DOI:10.1097/LVT.0000000000000172] [PMID]
- Wu G, Wu D, Lo J, Wang Y, Wu J, Lu S, et al. A bioartificial liver support system integrated with a DLM/GelMA-based bioengineered whole liver for prevention of hepatic encephalopathy via enhanced ammonia reduction. Biomaterials Science. 2020; 8(10):2814-24. [DOI:10.1039/C9BM01879D] [PMID]
- Segura-Aguilar J, Mannervik B, Inzunza J, Varshney M, Nalvarte I, Muñoz P. Astrocytes protect dopaminergic neurons against aminochrome neurotoxicity. Neural Regeneration Research. 2022; 17(9):1861-6. [DOI:10.4103/1673-5374.335690] [PMID] [PMCID]
- Claeys W, Van Hoecke L, Geerts A, Van Vlierberghe H, Lefere S, Van Imschoot G, et al. A mouse model of hepatic encephalopathy: Bile duct ligation induces brain ammonia overload, glial cell activation and neuroinflammation. Scientific Reports. 2022; 12(1):17558. [DOI:10.1038/s41598-022-22423-6] [PMID] [PMCID]
- Li Y, Pan L, Zeng X, Zhang R, Li X, Li J, et al. Ammonia exposure causes the imbalance of the gut-brain axis by altering gene networks associated with oxidative metabolism, inflammation and apoptosis. Ecotoxicology and Environmental Safety. 2021; 224:112668. [DOI:10.1016/j.ecoenv.2021.112668] [PMID]
- Kerbert AJ, Engelmann C, Jalan R. Neurocritical care management of hepatic encephalopathy and coma in liver failure. Seminars in Respiratory and Critical Care Medicine. 2018; 39(5):523-37. [DOI:10.1055/s-0038-1672180] [PMID]
- Ferenci P, Lockwood A, Mullen K, Tarter R, Weissenborn K, Blei AT. Hepatic encephalopathy--definition, nomenclature, diagnosis, and quantification: Final report of the working party at the 11th World Congresses of Gastroenterology, Vienna, 1998. Hepatology. 2002; 35(3):716-21. [DOI:10.1053/jhep.2002.31250] [PMID]
- Weller M, Le Rhun E, Van den Bent M, Chang SM, Cloughesy TF, Goldbrunner R, et al. Diagnosis and management of complications from the treatment of primary central nervous system tumors in adults. Neuro-oncology. 2023; 25(7):1200-24. [DOI:10.1093/neuonc/noad038] [PMID] [PMCID]
- Tranchita E, Cafiero G, Giordano U, Palermi S, Gentili F, Guzzo I, et al. Differences in physical activity levels between healthy and transplanted children: Who needs more tips? Healthcare. 2023; 11(11):1610. [DOI:10.3390/healthcare11111610] [PMID] [PMCID]
- Karam V, Castaing D, Danet C, Delvart V, Gasquet I, Adam R, et al. Longitudinal prospective evaluation of quality of life in adult patients before and one year after liver transplantation. Liver Transplantation. 2003; 9(7):703-11. [DOI:10.1053/jlts.2003.50148] [PMID]
- Fasihi L, Tartibian B, Eslami R, Fasihi H. Artificial intelligence used to diagnose osteoporosis from risk factors in clinical data and proposing sports protocols. Scientific Reports. 2022; 12(1):18330. [DOI:10.1038/s41598-022-23184-y] [PMID] [PMCID]
- Moghadam MC, Abad EMK, Bagherzadeh N, Ramsingh D, Li GP, Kain ZN. A machine-learning approach to predicting hypotensive events in ICU settings. Computers in Biology and Medicine. 2020; 118:103626. [DOI:10.1016/j.compbiomed.2020.103626] [PMID]
- Pepin KJ, Cook EF, Cohen SL. Risk of complication at the time of laparoscopic hysterectomy: A prediction model built from the national surgical quality improvement program database. American Journal of Obstetrics and Gynecology. 2020; 223(4):555.e1-555. [DOI:10.1016/j.ajog.2020.03.023] [PMID]
- Merath K, Hyer JM, Mehta R, Farooq A, Bagante F, Sahara K, et al. Use of machine learning for prediction of patient risk of postoperative complications after liver, pancreatic, and colorectal surgery. Journal of Gastrointestinal Surgery. 2020; 24(8):1843-51. [DOI:10.1007/s11605-019-04338-2] [PMID]
- Zeng S, Li L, Hu Y, Luo L, Fang Y. Machine learning approaches for the prediction of postoperative complication risk in liver resection patients. BMC Medical Informatics and Decision Making. 2021; 21(1):371. [DOI:10.1186/s12911-021-01731-3] [PMID] [PMCID]
- Fasihi L, Tartibian B, Eslami R. [Comparing the effectiveness of two simple decision tree and random forest algorithms in predicting osteoporosis in active middle-aged men (Persian)]. The Scientific Journal of Rehabilitation Medicine. 2022; 11(2):264-75. [DOI:10.32598/SJRM.11.2.10]
- Fasihi L, Tartibian B, Eslami, R. [Presenting a model for detecting osteoporosis in active older men using the support vector machine algorithm (Persian)]. The Scientific Journal of Rehabilitation Medicine. 2022; 11(5):742-53. [DOI:10.32598/SJRM.11.5.8]
- Tartibian B, Fasihi L, Eslami R. Prediction of osteoporosis by k- nn algorithm and prescribing physical activity for elderly women. New Approaches in Exercise Physiology. 2020; 2(4):87-100. [DOI:10.22054/nass.2021.59130.1088]
- Shahraki MR, Mesgar M. [Evaluation of data mining algorithms for detection of liver disease (Persian)]. Payavard Salamat. 2019; 13(1):81-90. [DOI:10.5121/acij.2019.10501]
- Cruz-Ramírez M, Hervás-Martínez C, Fernández JC, Briceño J, de la Mata M. Predicting patient survival after liver transplantation using evolutionary multi-objective artificial neural networks. Artificial Intelligence in Medicine. 2013; 58(1):37-49. [DOI:10.1016/j.artmed.2013.02.004] [PMID]
- Waseem A, Jamal A, Qadir A, Amitabh V. Serum prolactin as a marker of the severity of liver cirrhosis in a tertiary hospital in India: A cross-sectional study. Nigerian Journal of Clinical Practice. 2024; 27(7):844-9. [DOI:10.4103/njcp.njcp_880_23] [PMID]
- Fernández-Delgado M, Cernadas E, Barro S, Amorim D. Do we need hundreds of classifiers to solve real world classification problems? The Journal of Machine Learning Research. 2014; 15(1):3133-81. [DOI: 10.1016/ jmlr.2014.3452]
- Bosoi CR, Oliveira MM, Ochoa-Sanchez R, Tremblay M, Ten Have GA, Deutz NE, et al. The bile duct ligated rat: A relevant model to study muscle mass loss in cirrhosis. Metabolic Brain Disease. 2017; 32(2):513-8. [DOI:10.1007/s11011-016-9937-4] [PMID]
- Wilborn CD, Taylor LW, Greenwood M, Kreider RB, Willoughby DS. Effects of different intensities of resistance exercise on regulators of myogenesis. Journal of Strength and Conditioning Researchs. 2009; 23(8):2179-87. [DOI:10.1519/JSC.0b013e3181bab493] [PMID]
- Hayashi F, Matsumoto Y, Momoki C, Yuikawa M, Okada G, Hamakawa E, et al. Physical inactivity and insufficient dietary intake are associated with the frequency of sarcopenia in patients with compensated viral liver cirrhosis. Hepatology Research. 2013; 43(12):1264-75. [DOI:10.1111/hepr.12085] [PMID]